Neeraj Singh, a leading figure in Information Technology and Security, is currently the Head of IT & Information Security at InterGlobe Enterprises. With over two decades of experience, Neeraj excels in product development, strategic IT management, and state-of-the-art security practices. His expertise covers network security, data protection, risk assessment, and cybersecurity strategy. Neeraj’s leadership fortifies InterGlobe’s digital infrastructure, ensuring confidentiality in the digital landscape. His role emphasizes innovation and growth. Neeraj holds prestigious certifications such as CCISO, CISM, CISA, PMP, and ITIL, underscoring his commitment to excellence in the ever-evolving IT and security field.
In a privileged conversation with The Interview World, Neeraj highlights the significant impact of AI on the cybersecurity landscape and underscores the critical need for proactive measures to protect enterprises from the increasing wave of cyber threats. The following excerpts provide key insights from the interview.
Q: How has artificial intelligence transformed the landscape of cybersecurity in recent years, and what key advantages does AI bring to this field?
A: Artificial intelligence (AI) has exerted a profound influence on the cybersecurity landscape in recent years, and it continues to play a pivotal role in bolstering organizations’ defences against ever-evolving cyber threats. AI has brought about a revolution in the field of cybersecurity by bolstering threat detection and response capabilities. By harnessing machine learning, AI meticulously scrutinizes vast datasets to pinpoint anomalies, leading to expedited cyber threat identification. Furthermore, it enhances the precision of threat classification while minimizing false alarms, enabling security teams to focus their attention on legitimate threats. Additionally, AI streamlines the execution of routine tasks, reducing the burden on cybersecurity professionals and empowering them to concentrate on more intricate challenges. AI-driven technologies have the capacity to adapt and evolve in response to emerging threats, rendering them invaluable assets for safeguarding digital resources.
However, while AI offers numerous advantages in the realm of cybersecurity, it is not a panacea and should be employed in conjunction with other security measures. Cybersecurity constitutes an ongoing and multifaceted challenge that necessitates a combination of technology, processes, and highly skilled professionals to effectively fortify defences against threats.
Q: What are the most common cybersecurity threats that AI can help detect or mitigate, and how does it do so effectively?
A: AI can effectively help detect and mitigate a wide range of cybersecurity threats, including:
- Malware and Ransomware: AI analyses the file and network behaviour for patterns indicative of known and new malware. It can detect and prevent these threats in real-time, even when specific signatures are unknown.
- Phishing Attacks: AI analyses emails and contextual information to identify phishing attempts by recognizing malicious content, sender behaviour, and deceptive techniques.
- Insider Threats: AI monitors user and entity behaviour, identifying unusual activities that may indicate insider threats, compromised accounts, or data exfiltration.
- DDoS Attacks: AI detects distributed denial of service (DDoS) attacks by analysing network traffic for anomalies, and identifying sudden traffic spikes and patterns associated with DDoS activity.
- Zero-Day Exploits: AI identifies previously unknown vulnerabilities and threats by detecting abnormal behaviour and deviations from normal system activity.
- Advanced Persistent Threats (APTs): AI continuously monitors network and endpoint activities to detect persistent, stealthy, and targeted attacks, identifying indicators of compromise and tactics used by APT actors.
- Credential Stuffing and Brute-Force Attacks: AI detects login attempts with unusual patterns or excessive failures, helping protect against account takeovers.
- Web Application Attacks: AI-enhanced Web Application Firewalls (WAFs) can analyse incoming traffic to block SQL injection, cross-site scripting (XSS), and other application-layer attacks.
- Data Exfiltration: AI monitors data flows and user behaviour, identifying unusual data access or transfer patterns that might indicate data exfiltration attempts.
- IoT Device Vulnerabilities: AI continuously assesses IoT device behaviour for vulnerabilities, abnormal device activity, and potential security risks, including compromised IoT devices within a network.
AI excels in threat detection and mitigation, leveraging its capacity to swiftly process vast datasets in real-time, identify both patterns and anomalies and adapt to emerging threats. This technology has the potential to substantially diminish false positives, bolstering organizations’ security postures through timely alerts and automated threat responses.
Q: Could you explain the role of machine learning in threat detection and prevention? What are some specific ML techniques commonly used in cybersecurity?
A: Machine learning (ML) plays a critical role in threat detection and prevention in cybersecurity by automating the analysis of vast datasets to identify and respond to security threats. Common ML techniques used in cybersecurity include:
- Anomaly Detection: ML models identify abnormal patterns or behaviour in data, helping to detect unknown or emerging threats.
- Signature-Based Detection: ML compares incoming data against known patterns or signatures of known threats, such as malware or viruses.
- Behavioural Analysis: ML monitors user and system behaviour to detect deviations from normal patterns, aiding in the identification of insider threats and unusual activities.
- Predictive Analysis: ML leverages historical data to forecast potential threats, enabling proactive security measures.
- Natural Language Processing (NLP): NLP techniques analyse text data, such as emails, to identify phishing attempts, malicious content, and suspicious communication.
- Image Analysis: ML is used to analyse images and videos for security purposes, such as monitoring surveillance camera feeds.
- Clustering and Classification: ML algorithms group data into categories, helping in the classification of network traffic, malware, and security incidents.
- Decision Trees and Random Forests: These models make decisions based on data, often used in intrusion detection and classification tasks.
- Deep Learning: Deep neural networks, including Convolutional Neural Networks (CNNs) and Recurrent Neural Networks (RNNs), are used for image analysis, malware detection, and natural language processing.
ML techniques enhance threat detection by processing large volumes of data in real time, identifying patterns, and adapting to evolving threats, which reduces false positives and enhances overall cybersecurity.
Q: One challenge in AI-based cybersecurity is dealing with false positives and false negatives. How do experts address this issue, and what strategies can be used to improve accuracy?
A: Dealing with false positives and false negatives in AI-based cybersecurity is a significant challenge, as both can have serious consequences. False positives can lead to unnecessary alerts and operational overhead, while false negatives can allow real threats to go undetected. Cybersecurity experts use various strategies to address this issue and improve accuracy:
- Fine-tuning: Continuously fine-tune machine learning models by adjusting parameters and retraining with updated data to minimize errors.
- Feature Engineering: Carefully select and engineer the right features to reduce noise and improve model accuracy.
- Hybrid Approaches: Combine multiple detection techniques to cross-verify alerts and reduce errors, providing a more comprehensive view of the security landscape.
- Contextual Analysis: Consider additional information about events and activities to better assess the validity of alerts, such as user behaviour or network context.
- Threshold Adjustment: Fine-tune alert thresholds to strike a balance between sensitivity and specificity, minimizing false positives without compromising threat detection.
- Feedback Loops: Use feedback from alerts and incidents to retrain models and improve accuracy over time.
- Ensemble Learning: Combine multiple models’ outputs using ensemble techniques to reduce errors and enhance accuracy.
- Threat Intelligence: Incorporate threat intelligence feeds and IoCs to provide context for assessing alert validity.
- Behavioural Analysis: Implement user and entity behaviour analysis (UEBA) to create baselines of normal behaviour, improving accuracy in detecting deviations.
- Regular Testing and Validation: Continuously test system performance through red teaming, penetration testing, and benchmark datasets to identify vulnerabilities and areas for improvement.
- Human Oversight: Skilled security analysts provide oversight and validate AI-generated alerts, ensuring informed decisions to confirm or reject alerts.
- Continuous Learning: Stay updated with the latest attack techniques and adjust AI systems accordingly in response to evolving threats.
These strategies, combined with a balance between detection and false alarms, are crucial for effectively protecting against threats while minimizing operational disruptions.
Q: Adversarial attacks on AI models have gained attention recently. How do these attacks work, and what measures can organizations take to defend against them in the context of cybersecurity?
A: Adversarial attacks manipulate input data to deceive AI models, causing incorrect outputs. Attackers use slight, imperceptible changes in data to create adversarial examples. These attacks exploit vulnerabilities in AI models, particularly deep neural networks. Measures to defend against them include:
- Adversarial Training: Re-train models using adversarial examples, making them more robust against such attacks.
- Robust Optimization: Modify optimization algorithms to account for adversarial examples during training.
- Input Preprocessing: Apply techniques like feature squeezing to remove subtle adversarial perturbations from data.
- Ensemble Learning: Combine multiple models with diverse architectures to make crafting universal adversarial attacks more challenging.
- Regular Updates: Stay current with adversarial attack techniques and update defences accordingly.
- Human Oversight: Human experts can validate AI model outputs and help detect adversarial attacks.
- Threat Intelligence: Monitor and understand the evolving landscape of adversarial attacks.
- Regulatory Compliance: Ensure compliance with regulations like GDPR, which requires safeguarding AI models against adversarial attacks that may compromise data privacy.
Defending against adversarial attacks requires a multi-faceted approach, combining technical defences with human expertise and compliance measures. It is an evolving challenge, demanding vigilance and adaptability to address new attack techniques.
Q: AI can enhance security but also be vulnerable to exploitation. What safeguards should organizations put in place to protect their AI-based cybersecurity systems from attacks?
A: To protect their AI-based cybersecurity systems from attacks, organizations should implement a range of safeguards and security measures. These safeguards can help enhance the security and resilience of AI systems while reducing the risk of exploitation. Here are key measures organizations should consider:
- Data Security: Implement strong data encryption, access controls, and data loss prevention measures.
- Model Security: Secure AI models using trusted execution environments or secure containers.
- Adversarial Attack Mitigation: Detect and mitigate adversarial attacks using techniques like adversarial training.
- Access Controls: Enforce strict access controls and multi-factor authentication.
- Monitoring and Anomaly Detection: Continuously monitor AI system behaviour and apply anomaly detection algorithms.
- Incident Response Plan: Develop a comprehensive incident response plan tailored to AI-based systems.
- Regular Updates: Keep AI components up to date to address vulnerabilities.
- Redundancy and Failover: Implement redundancy and failover mechanisms to ensure availability.
- Employee Training: Educate employees on security best practices and awareness.
- Security Audits: Conduct regular security audits and penetration tests.
- Vendor and Supply Chain Security: Assess third-party vendors’ security practices.
- Regulatory Compliance: Adhere to relevant regulations and compliance requirements.
- Secure Development: Implement secure coding practices and ethical guidelines.
Safeguarding AI systems is an ongoing effort requiring a combination of technical, procedural, and organizational measures to harness AI’s benefits while minimizing risks.
Q: Can you provide examples of successful AI-driven cybersecurity implementations or case studies where AI significantly improved a company’s security posture?
A: Certainly, here are a couple of examples of successful AI-driven cybersecurity implementations:
Darktrace at Maersk: In the aftermath of the NotPetya ransomware attack, Maersk, a global shipping company, implemented Darktrace’s AI-based cybersecurity platform. Darktrace’s system continuously analysed network traffic, user behaviour, and device activities. During a subsequent ransomware attack attempt, Darktrace detected the intrusion and automatically stopped the attack, preventing a potentially devastating incident. Maersk credited Darktrace with its ability to respond swiftly, minimizing the impact on its operations.
JPMorgan Chase’s COIN: JPMorgan Chase developed COIN (Contract Intelligence), an AI-driven system for reviewing and extracting insights from its vast legal documents. This AI solution enabled the bank’s legal team to review contracts more efficiently and accurately. By automating contract analysis, JPMorgan reduced the time and resources needed for the task, improved compliance, and lowered the risk of missing critical details in legal agreements. In both cases, AI played a crucial role in enhancing security and operational efficiency. Maersk leveraged AI for real-time threat detection, while JPMorgan used AI for contract analysis and compliance. These implementations showcase the versatility and effectiveness of AI in addressing security and operational challenges.
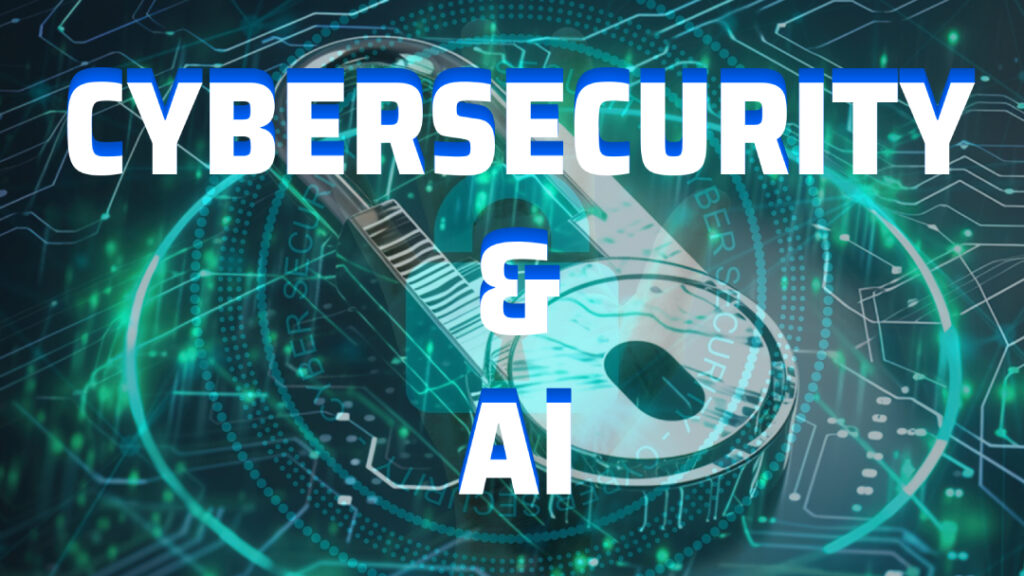