The evolution of AI agents signals a radical shift—from rigid, rule-based automation to autonomous, context-aware systems that can reason, learn, and make decisions independently. What began as narrow-task executors has now matured into sophisticated, multimodal agents. These agents proactively engage across diverse formats—text, voice, images, and even video—delivering highly personalized, seamless experiences.
Today’s most disruptive trend lies in the emergence of agentic workflows. Here, AI agents not only collaborate with humans but also with each other to execute multi-step, goal-oriented tasks. With large language models (LLMs) at their core, these agents now comprehend natural language with remarkable depth. As a result, they effortlessly interpret instructions, retrieve relevant information, and carry out complex operations.
Meanwhile, significant strides in memory architecture and tool-use enable agents to navigate dynamic environments and deliver contextually nuanced outputs. The rise of open-source frameworks and orchestration platforms is further democratizing access to these technologies. Yet, as their capabilities expand, so does the need for ethical design and explainability—both critical to ensuring trust and accountability.
As AI agents grow more intelligent and autonomous, they stand ready to redefine how industries drive productivity, unlock creativity, and enhance strategic decision-making.
In an exclusive interview with The Interview World, Navdeep Agarwal, Head of Business Intelligence at Americana Restaurants, sheds light on this transformative frontier. He explores how enterprises can integrate AI agents into their systems without disrupting workflows, how these agents ascend the decision-making ladder, and why reliability remains central. He also decodes the architecture behind complex AI agents and outlines how organizations should gear up for the next wave of AI-driven intelligence and deep analytics.
Here are the key takeaways from his thought-provoking conversation.
Q: AI agents are generating a lot of buzz lately—are they truly revolutionizing the way we approach analytics, or is it just hype?
A: We’ve moved beyond static dashboards and traditional reports. AI agents now act on data in real time. They detect anomalies, trigger alerts, and, in many cases, execute low-level decisions without human intervention. This marks a decisive shift—from passive analysis to autonomous action.
Q: With change happening so fast, how do companies manage to keep up?
A: Achieving success with AI agents begins with a deep understanding of the business at its most fundamental level. Organizations that can deconstruct their domain into core components gain a significant strategic advantage.
From this foundation, they can build effectively by adopting agent orchestration platforms to manage diverse types of AI agents tailored to specific functions. Introducing natural language interfaces allows business teams to interact directly with data, eliminating the need for technical intermediaries. The focus must shift from generating static reports to driving meaningful outcomes and actionable insights.
Crucially, organizations should start small—deploying a few high-impact use cases to demonstrate value before scaling with clarity and purpose.
Q: Integrating AI agents into enterprise systems sounds promising, but complex—how can organizations do it effectively without creating chaos?
A: Organizations must embed governance into their AI strategy from the very beginning. This means designing systems with robust safeguards, not as an afterthought but as a foundational principle. It starts with implementing semantic layers that abstract and protect sensitive data, followed by enforcing role-based and attribute-based access controls to ensure secure, compliant operations. Transparent data lineage and clear model explainability are equally critical—they build trust and accountability into the system.
Moreover, with today’s technology, enterprises can confidently build their entire AI agent stack within their own cloud environment, maintaining full control over infrastructure, data, and compliance. Just as important is the ethical dimension. Forward-thinking companies are now establishing dedicated AI Ethics Boards, recognizing that responsible innovation isn’t optional—it’s essential.
Q: With AI systems stepping into decision-making roles, how must enterprise analytics architectures evolve to keep pace and stay accountable?
A: The approach depends largely on an organization’s starting point. Those building from the ground up have a unique opportunity to design a context-aware, AI-native architecture tailored for agility and scale.
On the other hand, organizations with strong data foundations can immediately harness advanced capabilities—such as large language models, unstructured data processing, and deep learning—to accelerate impact. Regardless of the starting position, incorporating human-in-the-loop systems remains essential.
This not only ensures transparency and oversight but also maintains flexibility, allowing AI to operate effectively while humans guide critical decisions.
Q: As AI agents take on more analytical functions, how are the responsibilities of data engineers adapting to stay relevant?
A: AI agents are undergoing a rapid evolution, shifting from mere pipeline builders to powerful enablers of enterprise intelligence. Their responsibilities now extend far beyond data movement and integration. They manage embeddings and vector databases to optimize large language models (LLMs), and they construct semantic layers that enable agents to interpret and interact with data meaningfully.
Furthermore, they define and enforce data contracts to maintain consistency and integrity across systems. Internally, they establish self-service data marketplaces that democratize access and drive agility. By leveraging agentic platforms, they orchestrate complex automation workflows with precision. Increasingly, these agents operate alongside product teams, playing a more strategic role than ever before in shaping the organization’s AI-driven future.
Q: How do you ensure reliability in AI systems—especially when it comes to managing bias, data drift, and poor-quality inputs?
A: Several critical factors drive reliability in modern AI systems. High levels of digitization, reinforced by well-defined policies and data governance standards, lay a strong foundation for trustworthy data. However, reliability has evolved beyond structure alone. Today, context plays a pivotal role. Emerging tools such as vector databases, retrieval-augmented generation (RAG), and advanced prompting techniques enable AI to deliver results that are not only accurate but also contextually relevant. This marks a significant shift—a new layer of trust built on intelligent, situational awareness rather than static rules.
Q: With many enterprises shifting to hybrid and multi-cloud environments, what’s the optimal way to architect for AI in such complex setups?
A: Organizations must adopt a cloud-native approach while remaining cloud-agnostic—flexibility is non-negotiable. Building an AI-ready environment requires immediate action, especially as data now flows from a wide array of sources: internal systems, multiple cloud providers, on-premise infrastructure, and even public datasets.
To manage this complexity effectively, enterprises should implement Lakehouse architectures for unified and scalable data storage. Embracing data mesh or data fabric principles allows for decentralized ownership and seamless scalability. Workflow orchestration tools such as Dagster or Airflow ensure efficient data pipeline management. Just as critically, governance must operate across platforms to maintain consistency, compliance, and control.
Together, this infrastructure empowers organizations with the agility to scale and the oversight to stay in command.
Q: Looking ahead, what strategic priorities or disruptions should organizations be planning for in the AI and analytics space?
A: The critical shift begins with mindset. Organizations must reframe how they approach problems by fully leveraging today’s advanced capabilities. As Einstein once noted, imagination often surpasses knowledge—and in this era, it fuels innovation.
The next wave of transformation is already taking shape. AI-powered data engineering is becoming mainstream, with large language models assisting in everything from query generation to schema design and code development. Active metadata platforms, tightly integrated with semantic models, are redefining how data is organized and understood.
Contextual intelligence systems are emerging, capable of adapting in real time to dynamic environments. Meanwhile, zero-ETL architectures are eliminating the need for complex data pipelines, enabling direct, intelligent access to data. Together, these advancements mark the rise of truly intelligent data ecosystems—agile, adaptive, and profoundly transformative.
Q: With a whole new perspective emerging, are we witnessing a complete rewrite of the analytics playbook?
A: Undoubtedly, this shift is transformative. Teams that embrace it—rethinking their strategies through the lens of AI, contextual awareness, and emerging technologies—position themselves to lead the next wave of intelligent analytics. By proactively adapting, these organizations will not only stay ahead but also redefine the future of data-driven decision-making.
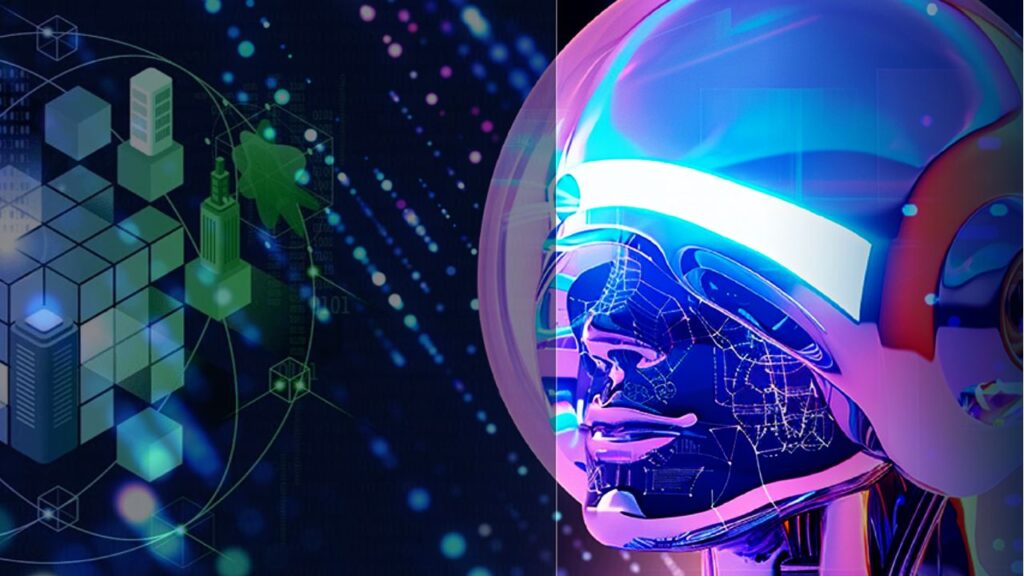